Author
stringlengths 6
28
| Birth Year
int64 1.85k
2k
| # of sitelinks
int64 0
190
| WikiData ID
stringlengths 5
9
| OpenLibrary ID
stringlengths 7
11
|
---|---|---|---|---|
Gabriel García Márquez | 1,927 | 190 | Q5878 | OL4586796A |
Toni Morrison | 1,931 | 122 | Q72334 | OL31120A |
Erich Maria Remarque | 1,898 | 119 | Q47293 | OL122169A |
Nadine Gordimer | 1,923 | 117 | Q47619 | OL20580A |
Isabel Allende | 1,942 | 91 | Q83566 | OL228079A |
Arundhati Roy | 1,961 | 85 | Q212801 | OL104867A |
Nikos Kazantzakis | 1,883 | 82 | Q214622 | OL29174A |
Oriana Fallaci | 1,929 | 73 | Q153700 | OL781814A |
Edith Stein | 1,891 | 71 | Q76749 | OL51184A |
Thomas Pynchon | 1,937 | 70 | Q35155 | OL4423376A |
Michael Ende | 1,929 | 69 | Q76498 | OL296646A |
Amin Maalouf | 1,949 | 69 | Q115243 | OL46671A |
Jean Dubuffet | 1,901 | 66 | Q170076 | OL143386A |
Julia Kristeva | 1,941 | 65 | Q159876 | OL31606A |
Joseph Heller | 1,923 | 64 | Q208101 | OL33512A |
Amos Oz | 1,939 | 64 | Q151872 | OL170730A |
Romain Gary | 1,914 | 63 | Q157322 | OL123692A |
Julia Child | 1,912 | 62 | Q214477 | OL218264A |
Pierre Boulez | 1,925 | 61 | Q156193 | OL273016A |
Mika Waltari | 1,908 | 60 | Q193111 | OL2688714A |
Gianni Rodari | 1,920 | 59 | Q193018 | OL299925A |
Thomas Bernhard | 1,931 | 58 | Q44336 | OL4326320A |
Manuel Azaña | 1,880 | 57 | Q203708 | OL149031A |
Christiaan Barnard | 1,922 | 57 | Q188803 | OL361761A |
Oskar Kokoschka | 1,886 | 54 | Q154260 | OL28175A |
James Hopwood Jeans | 1,877 | 54 | Q315545 | OL166245A |
David Foster Wallace | 1,962 | 53 | Q313246 | OL448939A |
Ivan Illich | 1,926 | 51 | Q84186 | OL428194A |
bell hooks | 1,952 | 50 | Q259507 | OL2631291A |
Kevin Smith | 1,970 | 49 | Q489831 | OL2721414A |
Carson McCullers | 1,917 | 47 | Q230591 | OL22420A |
Brendan Behan | 1,923 | 47 | Q313063 | OL143442A |
Peter Weiss | 1,916 | 46 | Q52191134 | OL396053A |
Marcel Aymé | 1,902 | 46 | Q318026 | OL75696A |
Olaf Stapledon | 1,886 | 45 | Q337373 | OL538087A |
Murray Bookchin | 1,921 | 45 | Q315910 | OL333834A |
Marianne Moore | 1,887 | 44 | Q278495 | OL545371A |
Veronica Roth | 1,988 | 43 | Q328212 | OL6895646A |
Leopoldo Alas | 1,852 | 43 | Q312747 | OL28169A |
Carl Zuckmayer | 1,896 | 43 | Q76820 | OL75772A |
Heinrich Harrer | 1,912 | 42 | Q84211 | OL207981A |
Frank McCourt | 1,930 | 42 | Q208869 | OL26363A |
David Suzuki | 1,936 | 42 | Q354534 | OL18944A |
Hermann Broch | 1,886 | 41 | Q84150 | OL61295A |
Richard Hammond | 1,969 | 40 | Q297265 | OL5572088A |
Maeve Binchy | 1,940 | 40 | Q152690 | OL21305A |
Ignazio Silone | 1,900 | 40 | Q168431 | OL124945A |
Herman Wouk | 1,915 | 40 | Q49072 | OL4352886A |
Eudora Welty | 1,909 | 40 | Q259364 | OL32584A |
Viktor Suvorov | 1,947 | 39 | Q130786 | OL284950A |
Knud Rasmussen | 1,879 | 39 | Q312769 | OL18679A |
Gary Snyder | 1,930 | 39 | Q315963 | OL22849A |
Frederick Jackson Turner | 1,861 | 39 | Q548462 | OL146604A |
Edith Nesbit | 1,858 | 39 | Q231708 | OL18053A |
Colm Tóibín | 1,955 | 38 | Q470758 | OL82249A |
Sarah Kane | 1,971 | 37 | Q231141 | OL1614632A |
Martin Andersen Nexø | 1,869 | 36 | Q168569 | OL137086A |
Timothy Garton Ash | 1,955 | 35 | Q311729 | OL81428A |
Nevil Shute | 1,899 | 35 | Q356639 | OL410117A |
Kostis Palamas | 1,859 | 35 | Q317967 | OL5868580A |
Fan S. Noli | 1,882 | 35 | Q366307 | OL46244A |
Arnold Wesker | 1,932 | 35 | Q202385 | OL22347A |
Daniel Ellsberg | 1,931 | 34 | Q431085 | OL1260683A |
Peter Shaffer | 1,926 | 33 | Q318188 | OL73801A |
Nancy Mitford | 1,904 | 33 | Q260026 | OL288327A |
Michael Ignatieff | 1,947 | 33 | Q311684 | OL235573A |
Leon Uris | 1,924 | 33 | Q269129 | OL19269A |
Gianni Vattimo | 1,936 | 33 | Q159648 | OL37112A |
Colin Dexter | 1,930 | 33 | Q457092 | OL34485A |
Pyotr Krasnov | 1,869 | 32 | Q35448 | OL188777A |
Linn Ullmann | 1,966 | 32 | Q256738 | OL31551A |
Ali Smith | 1,962 | 32 | Q468523 | OL6496199A |
Peter Atkins | 1,940 | 31 | Q369627 | OL3409121A |
Joanne Harris | 1,964 | 31 | Q234718 | OL25453A |
Dodie Smith | 1,896 | 31 | Q449085 | OL161177A |
Ernst Troeltsch | 1,865 | 30 | Q60285 | OL173237A |
Yu Hua | 1,960 | 28 | Q379520 | OL528199A |
Yrsa Sigurðardóttir | 1,963 | 28 | Q262253 | OL2631877A |
Stephen E. Ambrose | 1,936 | 28 | Q443953 | OL29987A |
Paolo Soleri | 1,919 | 28 | Q447351 | OL1123646A |
Guglielmo Ferrero | 1,871 | 28 | Q689713 | OL115322A |
Eric Temple Bell | 1,883 | 28 | Q548140 | OL766341A |
Cornelius Ryan | 1,920 | 28 | Q463975 | OL482577A |
Beverly Cleary | 1,916 | 28 | Q1316719 | OL22132A |
Karin Fossum | 1,954 | 27 | Q256789 | OL41672A |
John Perkins | 1,945 | 27 | Q465028 | OL1542161A |
Charles Fort | 1,874 | 27 | Q443325 | OL21506A |
Andre Gunder Frank | 1,929 | 27 | Q58040 | OL392296A |
William Beebe | 1,877 | 26 | Q956868 | OL155998A |
Murray Leinster | 1,896 | 26 | Q550449 | OL1232076A |
Mary Midgley | 1,919 | 26 | Q2898525 | OL448425A |
John Hersey | 1,914 | 26 | Q535812 | OL394640A |
Flora Nwapa | 1,931 | 26 | Q5460344 | OL2703239A |
Dietrich von Hildebrand | 1,889 | 26 | Q14678 | OL152394A |
Lauren Weisberger | 1,977 | 25 | Q176049 | OL1427597A |
Farley Mowat | 1,921 | 25 | Q966679 | OL30012A |
Ernst Haas | 1,921 | 25 | Q78767 | OL830687A |
August Derleth | 1,909 | 25 | Q509002 | OL6925253A |
Andrew Young | 1,932 | 25 | Q959635 | OL534748A |
Xiao Hong | 1,911 | 24 | Q464825 | OL1126811A |
Overview
🕮 KITAB is a challenging dataset and a dynamic data collection approach for testing abilities of Large Language Models (LLMs) in answering information retrieval queries with constraint filters. A filtering query with constraints can be of the form "List all books written by Toni Morrison that were published between 1970-1980"
. The dataset was originally contributed by the paper "KITAB: Evaluating LLMs on Constraint Satisfaction for Information Retrieval" Marah I Abdin, Suriya Gunasekar, Varun Chandrasekaran, Jerry Li, Mert Yuksekgonul, Rahee Ghosh Peshawaria, Ranjita Naik, and Besmira Nushi. 2023. The dataset is named after the word kitab, which is the word for "book" in Arabic, Swahili, Urdu, Hindi and various Indian and Turkic languages.
KITAB consists of book-related data across more than 600 authors and 13,000 queries with varying number of constraints and complexity. In each query in the dataset, the first constraint is always fixed to an author and the following can vary among the following types of book constraints to test for different constraint satisfaction capabilities:
- lexical (title starts or ends with a letter, word count in title)
- temporal (published between start and end year)
- named entity (city or human name present or not present in title)
What is available in this repository?
This repository contains the following artifacts:
- All data for the KITAB sample used in the original paper. This consists of the set of authors, their corresponding books, and the set of queries with constraints.
- Example code for generating a new sample with a different set of authors. Here the sampling and data collection steps do not include the generation of queries as these may change according to the evaluation usage needs for the data. The example code also shows how to evaluate a potential model output with a list of books against the provided ground truth in KITAB, by following the same evaluation process as in the original paper. Note that this evaluation tends to relax some of the constraint satisfaction requirements in particular when the model may come up with only a partial title.
- All prompts that were used in the original paper to evaluate GPT-4 and GPT-3.5.
Data
- KITAB-ONE-BOOK-CONSTRAINTS.json and KITAB-TWO-BOOK-CONSTRAINTS.json - correspond to queries with one and two book constraints. Each file has all the sufficient information that can be used to recreate a prompt query including the author, their birth year, number of sitelinks on WikiData, the constraint type(s), the constraint(s) expressed in natural language, the list of all books by the author, and the mapped list of books by the author that satisfy the constraint(s).
KITAB-ONE-BOOK-CONSTRAINTS_features = {
"Author": "author name",
"Birth Year": "author birth year",
"# of sitelinks": "number of external links related to the author",
"constraint_id": "unique id for the constraint",
"constraint_type": "type of the constraint",
"constraints": "the constraint",
"mapped_books": "list of books by the author mapped to the constraint",
"all_books": "full list of books by author post cleaning from openlibrary",
"raw_books": "raw list of books by author from openlibrary",
}
- KITAB-author-metadata.json - contains the set of 611 authors along with their birth year, the number of sitelinks in Wikidata, and their corresponding Open Library and WikiData identifiers.
- KITAB-book-metadata.tar.gz - contains a json file per author with all books retrieved from OpenLibrary for that author. The files contain the following information per title: the Open Library Id for the book, the Wikidata ID (if it exists), list of languages in which it was published, number of editions, number of words in the title, the earliest publishing year, city names found in the title (if any), a modified version of the title in lowercase that stripes stop words like "A" and "The" from the title, a set of of other redundant versions of the same title as found in Open Library (if any).
Code and evaluation scripts
Example notebooks included in this repository:
- collect_authors_from_wikidata.py and wikidata_open_library_author_profiling.ipynb - example code for generating a new author sample from WikiData and OpenLibrary. Here, we also make available the longer list of authors that was originally sampled from WikiData to facilitate the sampling process although future work may also choose to repeat this step as needed. The full list can be found in: wikidata_authors_crawl.csv.
- fetch_book_data.py - example code for collecting book data for the set of authors sampled in the previous steps. Pulls data from OpenLibrary and WikiData to curate and clean the sample.
- evaluation.ipynb - example code for evaluating model outputs from our prompts against ground truth KITAB data. Here, we also make available the GPT-4 output on human name detection, although as models improve future work may also choose to repeat this step as needed. Results can be found in: gpt_4_name_data_processed.csv.
Prompts
We use the following prompt templates for different experimental conditions on the KITAB data:
ALL-BOOKS (Template 1): List all books from the author. This condition enables us to estimate an upper bound of model performance in retrieving relevant information for all queries, regardless of other constraints.
NO-CONTEXT (Template 2a): List all books from the author that also satisfy other book constraints.
WITH-CONTEXT (Template 2b): First, provide a full list of books from the author as input context to the model. Then, ask the model to list all books from the author that also satisfy other book constraints.
SELF-CONTEXT (Template 3): Ask the model to first self-retrieve all books from the author, and then use that list to find those that also satisfy book constraints.
NAME-CHECK (Template 4): Ask the model to find all book in a given list that contain a human name.
Data Collection and Statistics
The author list was initially randomly sampled from WikiData and then filtered down to 611 authors to avoid potentially inaccurate data and extreme outliers. For example, this involved removing authors that have very few or too many books and authors that were born before 1850. The collected book data was derived from Open Library and contains all books from the author that are tagged to be in English by Open Library or detected to be in English by the Language Detection service from the Azure Cognitive Services API. More details about author sampling and book data collection and cleaning are present in the paper.
Since there exists a large number of constraint instances depending on their cardinality, we subsample from the potential large set of queries in a way that ensures a balanced representation across constraint types, and a variety of constraints that have different constrainedness (i.e., defined as the complement of the ratio between the number of books that satisfy the constraints with the total number of all books from the author). The dataset also contains “unsatisfiable” constraints, which do not match any book titles in our data. This constitutes 7.99% of the queries with only one book constraint. The final dataset contains 8239 single-constraint queries and 4750 double-constraint queries. The table below shows how these queries are distributed across different constraint types. For all double-constraint queries, both constraints are individually satisfiable and generated by combining our single constraint data. Only 0.76% of the queries are jointly unsatisfiable across both constraints.

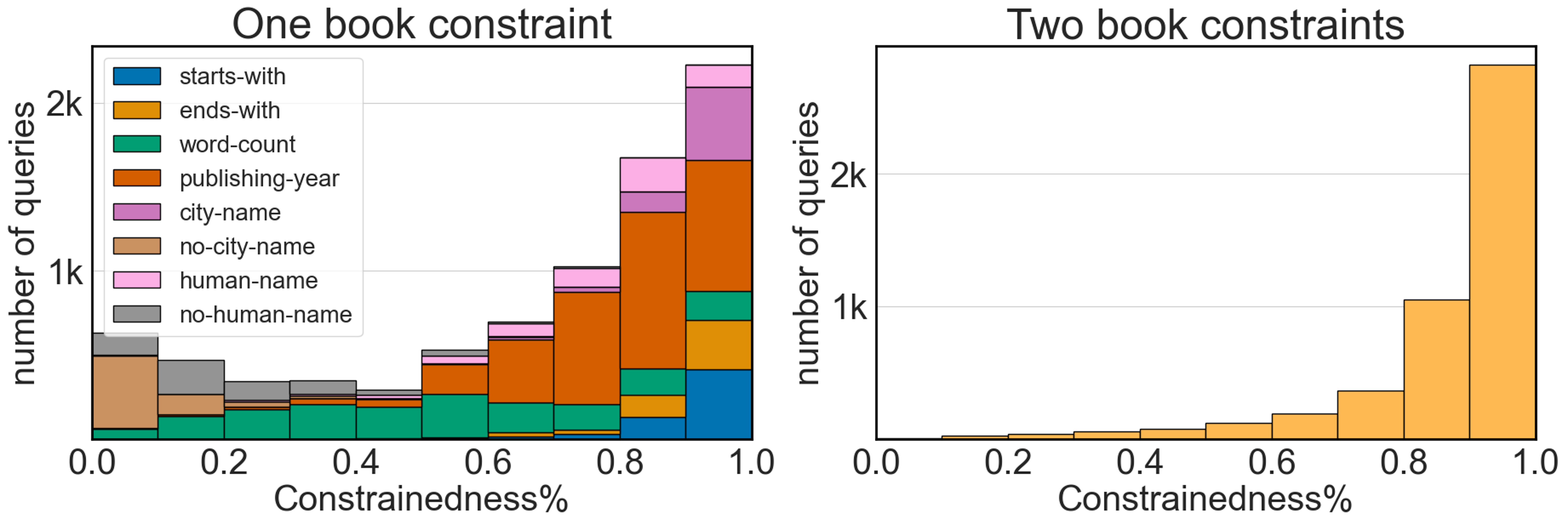
Responsible AI Considerations
Data Cleaning: Despite our best efforts in collecting a complete and accurate set of books, we also faced a variety of challenges in retrieval and cleaning, which we further describe in Appendix C.1 in the paper. To estimate the extent of which potential data cleaning issues may impact the data quality of KITAB and further evaluation, we also undertook a manual data annotation exercise during which we searched on the web for titles provided by GPT4 and GPT3.5 but that were marked as not from the author in our dataset. In summary, we find that based on a manual annotation of a subsample of queries, less than 5% of the queries to GPT4 and less than 6% of the queries to GPT3.5 may potentially be affected by cases where the model finds a book title that is not in KITAB and that will consequentially be marked as not from the author during our evaluation. While this can be remediated by using further data sources, the impact of missing information on model comparison is minor.
Human Names: Entity recognition for human names was done using both Azure Cognitive Services API and GPT4 (Template 4 in Appendix D in the paper), as we found the two approaches to be complementary for detecting names from different cultures. Note that even after using both these resources, there may still be names that are not recognized by either of these APIs, which is a testimony that more work is required in improving the quality of service of entity recognition for fairness across different languages and cultures.
City Names: For city names, we use Azure Cognitive Services API along with Geonames, a database of cities with more than 1000 inhabitants.
Author representation: The list of authors in KITAB was sampled randomly from a large set of authors present in Open Library. We see that the rate of irrelevant information generated by current models increases with a lower number of sitelinks in Wikidata. Since the number of sitelinks may also correlate with the age (birth year) of the author or even their nationality and how well their community is linked to the World Wide Web, this observation has important implications on model quality of service across different geographical regions and author popularity and age. While KITAB naturally does contain more authors with a lower number of sitelinks (as indicated by its long-tail distribution of author count vs. their popularity), future fairness measurement investigations in this regard may also need to oversample explicitly from cohorts belonging to given demographic and geographical attributes.
State-of-the-art results on KITAB
How to cite
@inproceedings{abdin2023kitab, title={KITAB: Evaluating LLMs on Constraint Satisfaction for Information Retrieval}, author={Abdin, Marah I and Gunasekar, Suriya and Chandrasekaran, Varun and Li, Jerry and Yuksekgonul, Mert and Peshawaria, Rahee Ghosh and Naik, Ranjita and Nushi, Besmira}, journal={arXiv preprint arXiv:2310.15511}, year={2023} }
Contributors
Marah I Abdin, Suriya Gunasekar, Varun Chandrasekaran, Jerry Li, Mert Yuksekgonul, Rahee Ghosh Peshawaria, Ranjita Naik, Besmira Nushi
- Downloads last month
- 169