Deploy LLama 2 in a few clicks on Inference Endpoints
Machine Learning At Your Service
With Inference Endpoints (dedicated), easily deploy Transformers, Diffusers or any model on dedicated, fully managed infrastructure. Keep your costs low with our secure, compliant and flexible production solution.
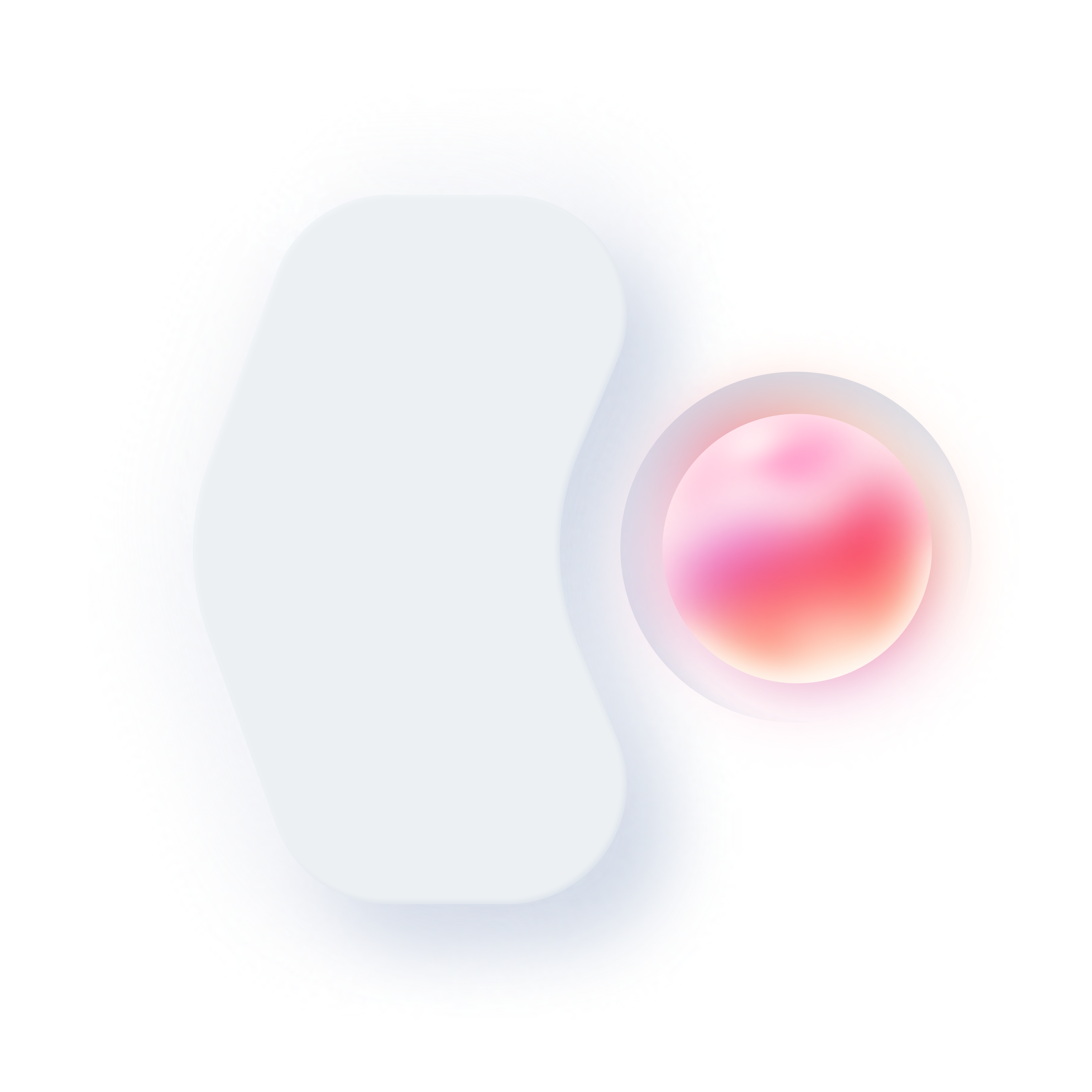
Production Inference Made Easy
Deploy models on dedicated and secure infrastructure without dealing with containers and GPUs
-
Deploy models with just a few clicks
- Turn your models into production ready APIs, without having to deal with infrastructure or MLOps.
-
Keep your production costs down
- Leverage a fully-managed production solution for inference and just pay as you go for the raw compute you use.
-
Enterprise Security
- Deploy models into secure offline endpoints only accessible via direct connection to your Virtual Private Cloud (VPCs).
How It Works
Deploy models for production in a few simple steps
1. Select your model
Select the model you want to deploy. You can deploy a custom model or any of the 60,000+ Transformers, Diffusers or Sentence Transformers models available on the π€ Hub for NLP, computer vision, or speech tasks.
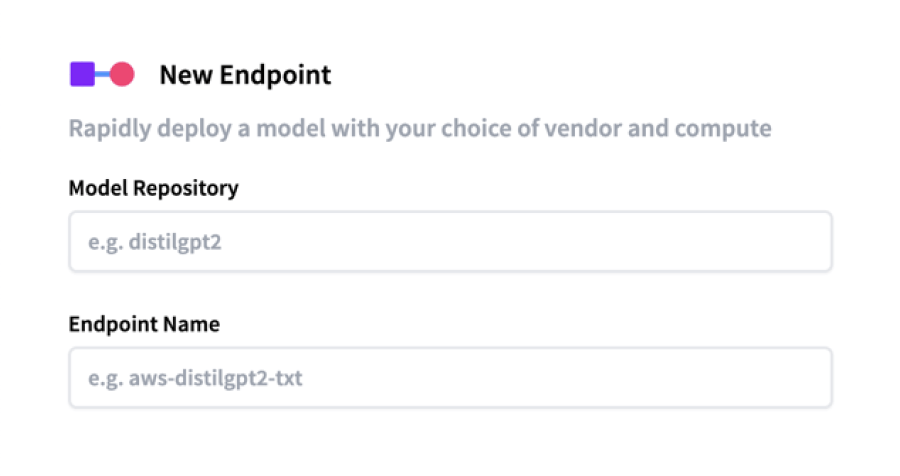
2. Choose your cloud
Pick your cloud and select a region close to your data in compliance with your requirements (e.g. Europe, North America or Asia Pacific).
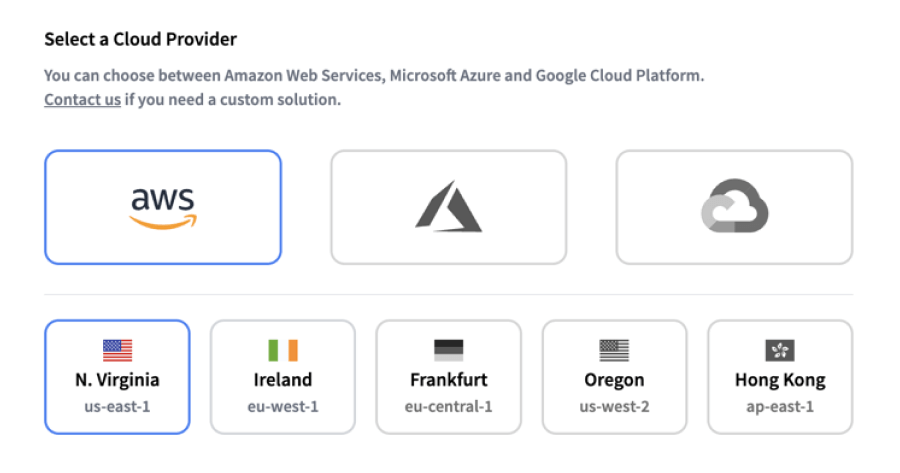
3. Select your security level
Protected Endpoints are accessible from the Internet and require valid authentication.
Public Endpoints are accessible from the Internet and do not require authentication.
Private Endpoints are only available through an intra-region secured AWS or Azure PrivateLink direct connection to a VPC and are not accessible from the Internet.
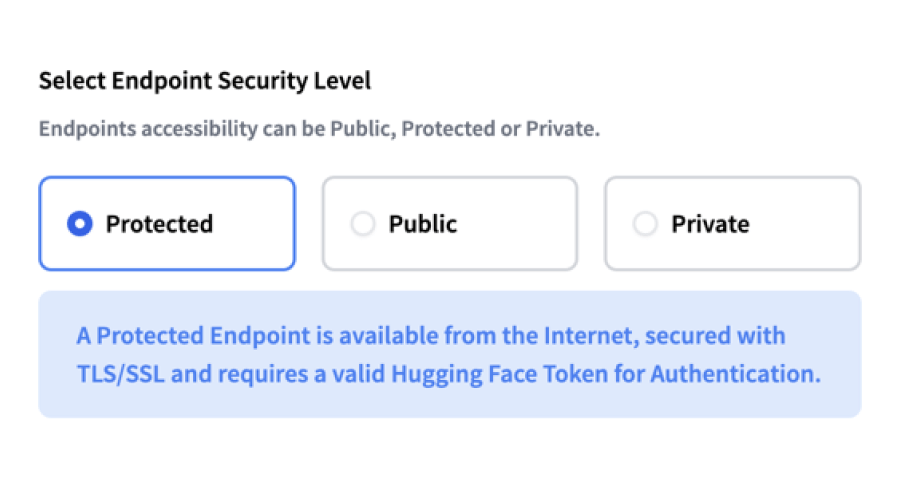
4. Create and manage your endpoint
Click create and your new endpoint is ready in a couple of minutes. Define autoscaling, access logs and monitoring, set custom metrics routes, manage endpoints programmatically with API/CLI, and rollback models - all super easily.
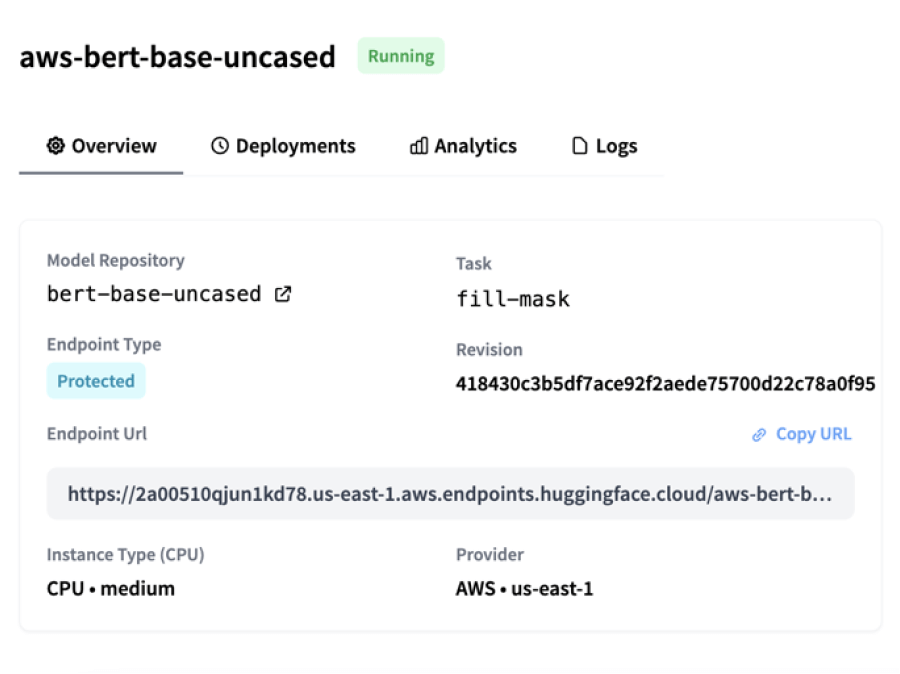
A Better Way to Go to Production
Scale your machine learning while keeping your costs low
Before
π€Ό
Struggle with MLOps and building the right infrastructure for production.
π’
Wasted time deploying models slows down ML development.
π
Deploying models in a compliant and secure way is difficult & time-consuming.
β
87% of data science projects never make it into production.
After
π€
Don't worry about infrastructure or MLOps, spend more time building models.
π
A fully-managed solution for model inference accelerates your ML roadmap.
π
Easily deploy your models in a secure and compliant environment.
β
Seamless model deployment bridges the gap from research to production.
Customer Success Stories
Learn how leading AI teams use π€ Inference Endpoints to deploy models
Endpoints for Music
Musixmatch is the worldβs leading music data company
Custom text embeddings generation pipeline
Distilbert-base-uncased-finetuned-sst-2-english
facebook/wav2vec2-base-960h
Custom model based on sentence transformers
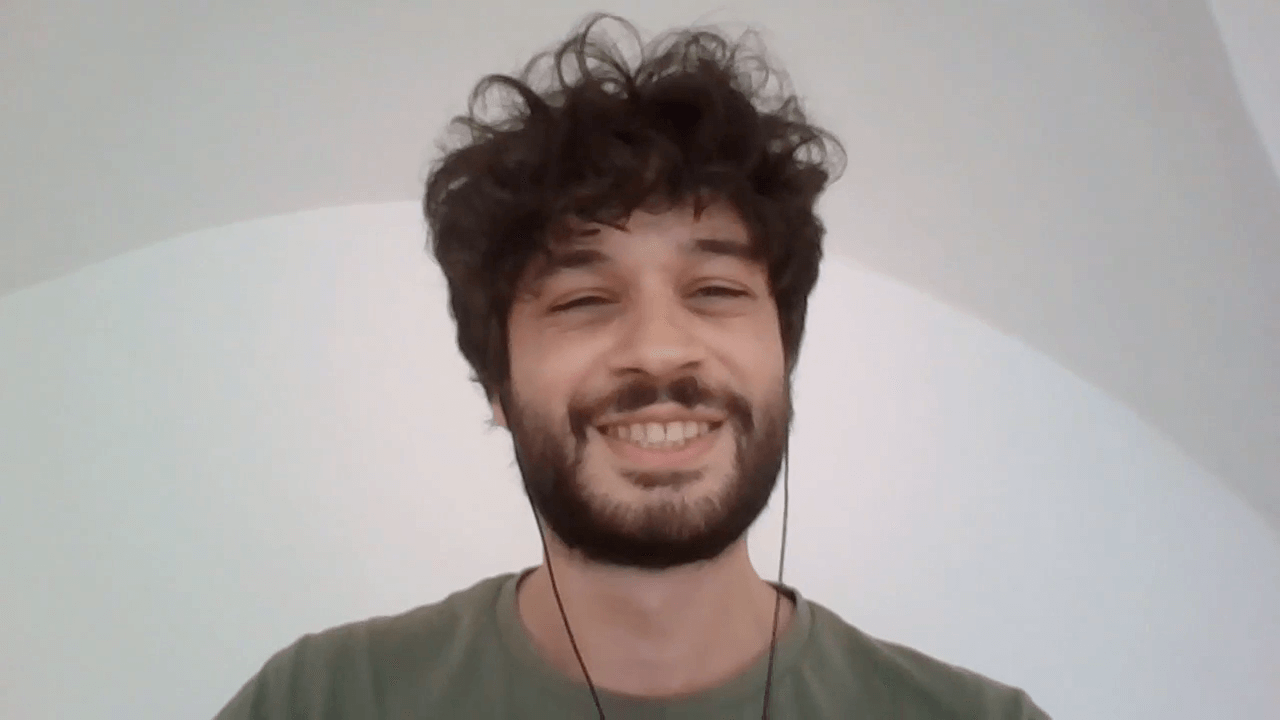
The coolest thing was how easy it was to define a complete custom interface from the model to the inference process. It just took us a couple of hours to adapt our code, and have a functioning and totally custom endpoint.
Endpoints for Health
Phamily improves patient health with intelligent care management
HIPAA-compliant secure endpoints for text classification
Custom model based on text-classification (MPNET)
Custom model based on text-classification (BERT)
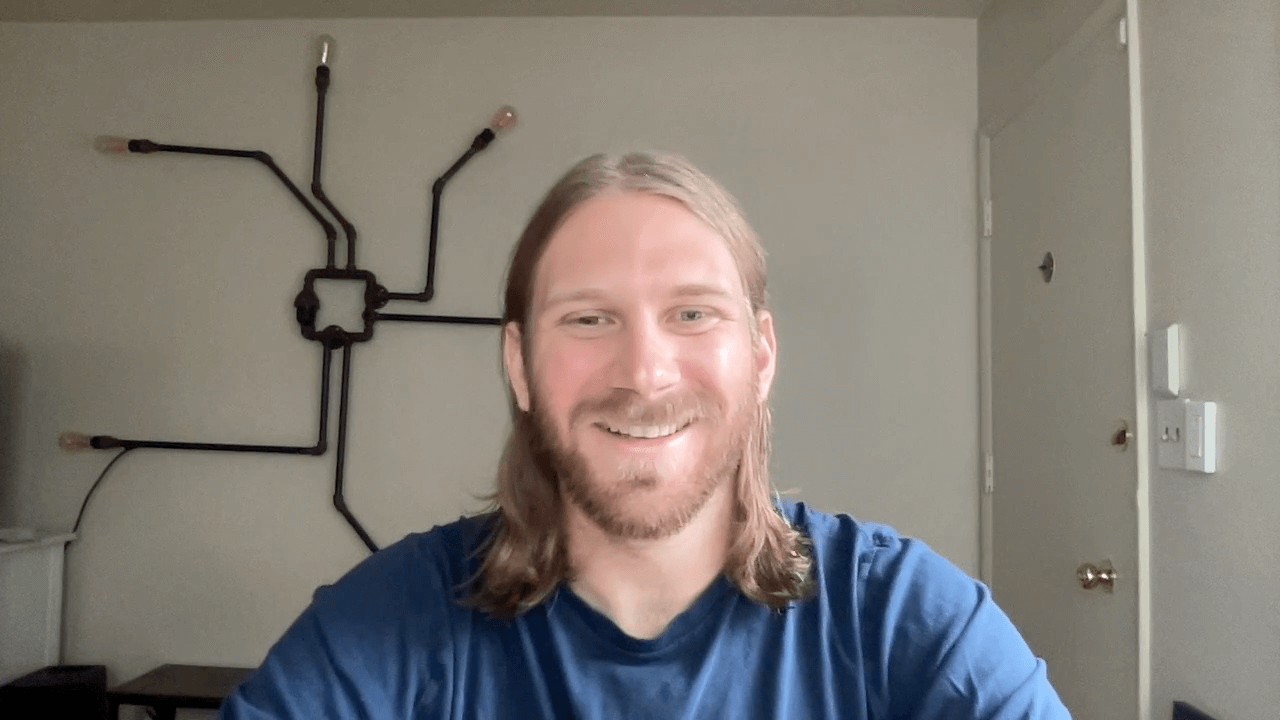
It took off a week's worth of developer time. Thanks to Inference Endpoints, we now basically spend all of our time on R&D, not fiddling with AWS. If you haven't already built a robust, performant, fault tolerant system for inference, then it's pretty much a no brainer.
Endpoints for Search
Pinecone is the vector database for intelligent search
Autoscaling endpoints for fast embeddings generation
Different sentence transformers and embedding models
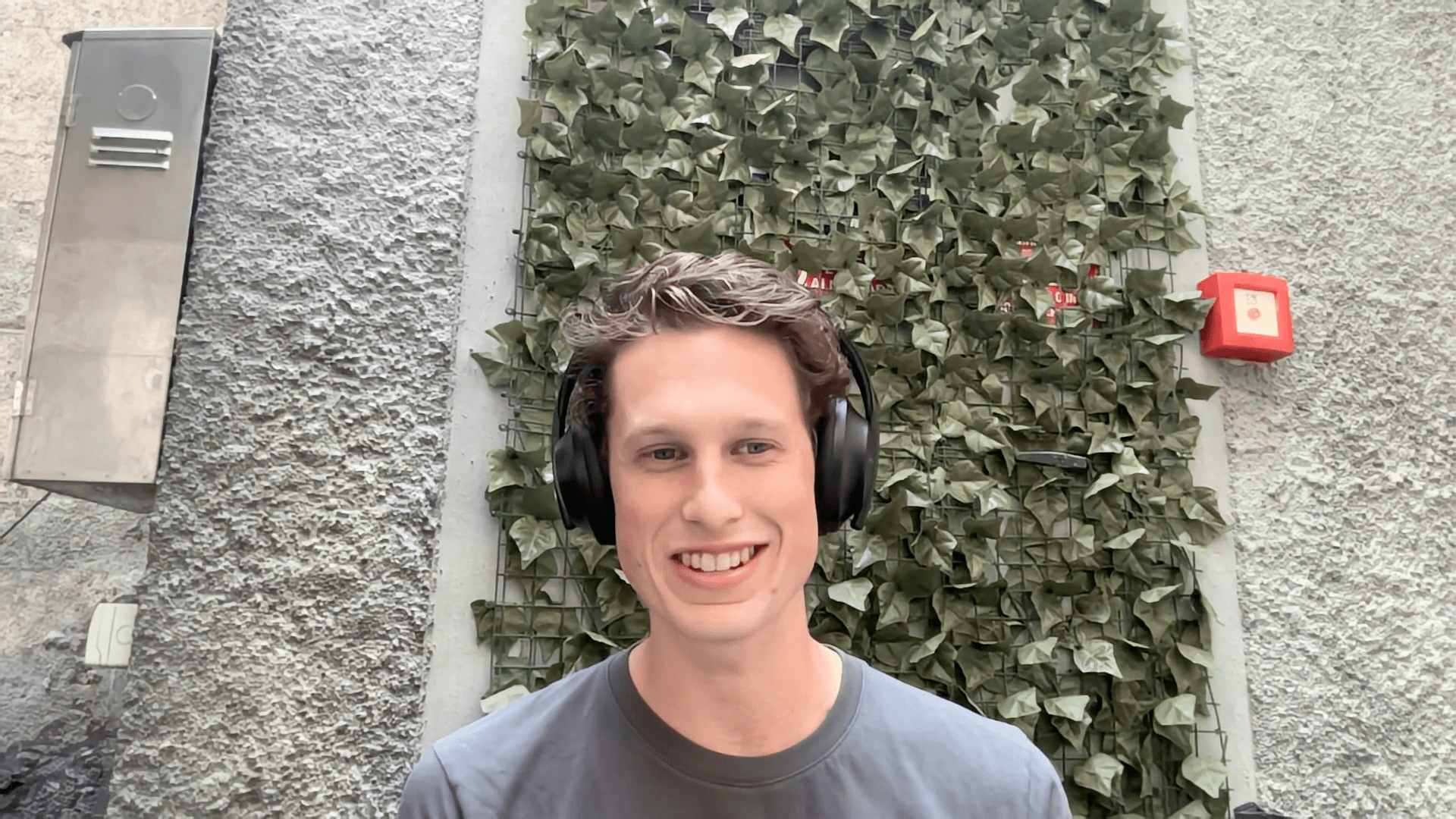
We were able to choose an off the shelf model that's very common for our customers to get started with and set it so that it can be configured to handle over 100 requests per second just with a few button clicks. With the release of the Hugging Face Inference Endpoints, we believe there's a new standard for how easy it can be to go build your first vector embedding based solution, whether it be semantic search or question answering system.
Endpoints for Videos
Waymark is a AI-powered video creator
Multi-modal endpoints for embeddings, audio and image generation
sentence-transformers/all-mpnet-base-v2
google/vit-base-patch16-224-in21k
Custom model based on florentgbelidji/blip_captioning
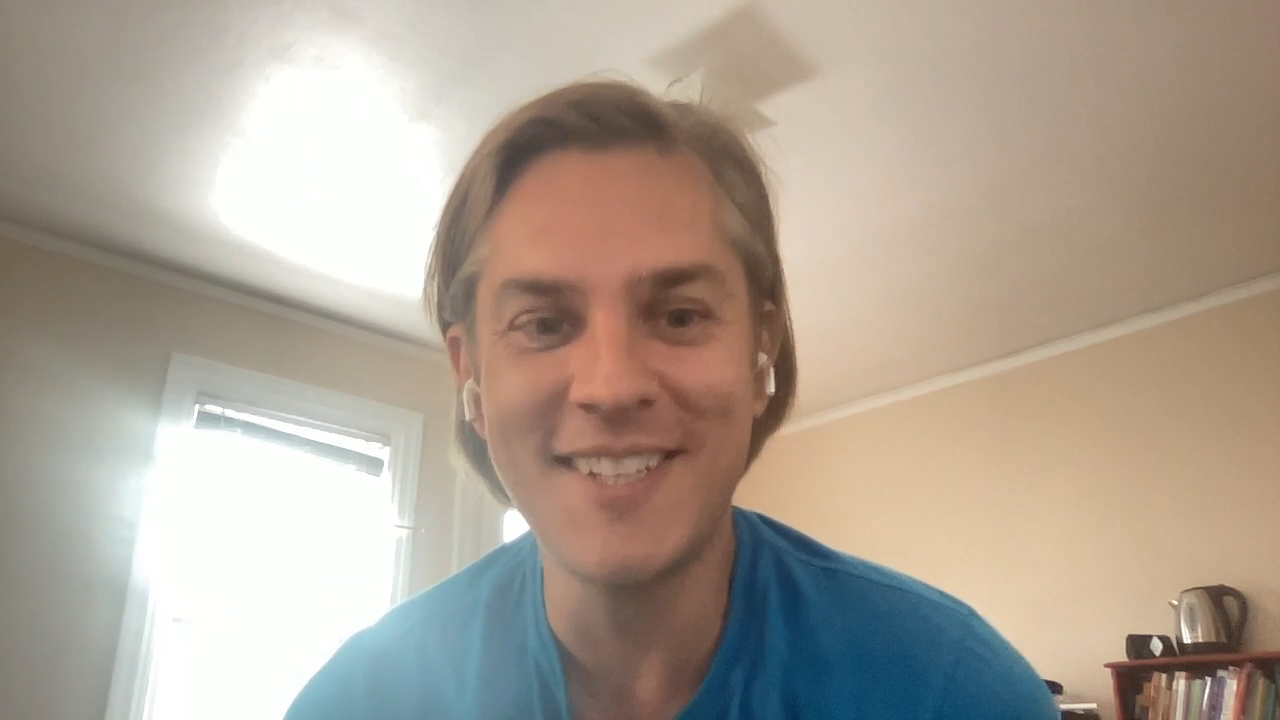
You're bringing the potential time delta between - I've never seen anything that could do this before - to - I could have it on infrastructure ready to support an existing product - down to potentially less than a day.
Pricing
Pay for CPU & GPU compute resources
π Self-serve
π’Enterprise
-
Inference Endpoints (dedicated)
Pay for compute resources uptime by the minute, billed monthly.
As low as $0.03 per CPU core/hr and $0.50 per GPU/hr.
-
Email Support
Email support and no SLAs.
-
Inference Endpoints (dedicated)
Custom pricing based on volume commit and annual contracts.
-
Dedicated Support & SLAs
Dedicated support, 24/7 SLAs, and uptime guarantees.
Start now with Inference Endpoints (dedicated)
Deploy models in a few clicks π€―
Pay for compute resources uptime, by the minute.